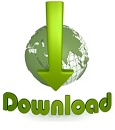
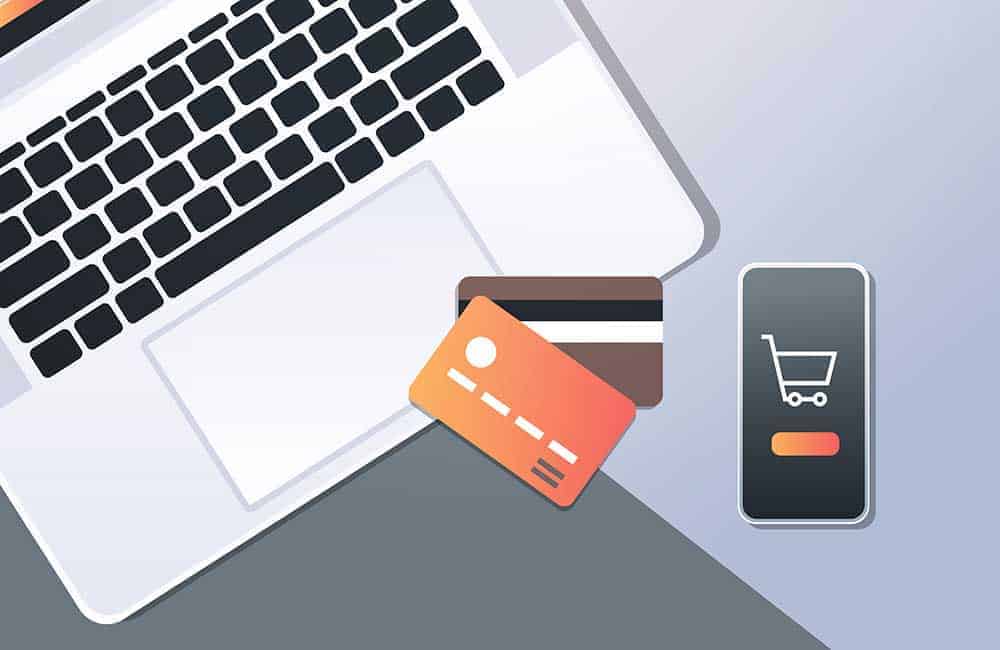
Alternatively, if the transaction has a very high probability of fraud, the Pub/Sub message could also automatically trigger and alert an internal anti-fraud team to flag and freeze the transaction. Then, any downstream service can actively listen to the Pub/Sub message and trigger notifications (for example, using Twilio) to confirm with the customer whether or not it was indeed them that initiated the transaction.

For example, if a transaction is predicted to be fraud with a probability of greater than 70%, then it should send a Pub/Sub message. We also want to make sure we can trigger some downstream action when fraud is detected. Setting up alert-based fraud notifications using Pub/Sub With the fraud detection Dataflow pipeline in place, the next step would be to set up fraud alerts.
CREDIT CARD TRANSACTION RISK ENGINE UPGRADE
Alternatively, if you still want to have the model hosted as its own endpoint, you can try to upgrade the machine type of the AI Platform model and increase the number of nodes ( documentation). First, instead of hosting the model on AI Platform, you can use a local version of the model saved on Dataflow workers and call it directly for prediction during the stream processing pipeline for each transaction, which can improve performance and reduce latency ( documentation). To decrease latency, there are a few ways. Quantiphi observes that the latency in this design pattern typically falls in line with customer requirements. With the auto-scaling functionality of Dataflow pipeline and the model deployed on Cloud AI Platform, Quantiphi found that the streaming dataflow pipeline takes a few seconds end-to-end from the transaction ingestion to outputting the ML prediction to both BigQuery (to maintain a record of predictions) and Pub/Sub (for downstream notifications). Below is an example of recent transactions for a particular credit card stored in Firestore.
CREDIT CARD TRANSACTION RISK ENGINE FULL
Of course, the full data is still stored in BigQuery. In other words, for each streaming transaction, the Dataflow pipeline does a lookup of the user's recent transactions on Firestore, computes the aggregations for that user (e.g., volume, frequency), and updates the Firestore record to only maintain the most recent transactions. In Firestore, by maintaining only the most recent transactions per user, we can easily perform quick lookups and compute aggregations as needed. To this end, we used Cloud Firestore, which is a flexible, scalable, serverless NoSQL cloud database designed to store and sync data for mobile, web and servers. In this step, we need to build a streaming Dataflow pipeline which consumes new transactions from a Pub/Sub subscription, invoke the ML models deployed on AI Platform and write the transactions along with model predictions to BigQuery.įor the derived features, we'll still need aggregate data computed in low latency. In other words, adding this step of model inference will allow us to detect fraud as soon as new incoming transactions are ingested in Dataflow.īuilding the streaming real time inference pipeline using Cloud Dataflow With the model working, we can now add this to the streaming Dataflow pipeline. In the case of credit card transactions, a Dataflow pipeline can ingest real-time data continuously and automatically scales based on the transaction volume without human involvement. In the following CREATE MODEL statement, XGBoost is trained to predict is_fraud (1 if fraud, else 0) based on various features like transaction category, transaction amount and demographics.ĭataflow can be used for both real-time predictions and batch inferences. Note that with BigQuery ML, you don't have to worry about spinning up any infrastructure - BigQuery handles that for you so you can focus on building and using the model.

Using the view from the previous section, you can train the model with just a few lines of code. You can choose from a variety of classification algorithms, including logistic regression, XGBoost, deep neural networks, or even AutoML Tables to have Google Cloud automatically search and create the best model for you.įor this pattern, we opted for the XGBoost model, which worked really well because it didn't require much hyperparameter tuning to perform well, and it still retained some level of model explainability. With both transactional data and customer demographics data in BigQuery, we can train a model using SQL with BigQuery ML.
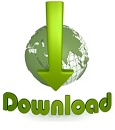